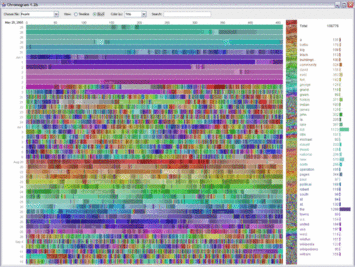
Worship at the altar of what is labelled big data is rampant in both corporate and large not-for-profit settings. And while there is some general sense that big data arrives at warp-speed and involves huge datasets from very diverse sources and methodologies, there is no consensus and little discussion of what comprises meaningful and valid “big” datasets.
It is part of the American DNA that that bigger means better, so the alchemy of big data can appear enticing. Moreover, big data naturally appeals to many data geeks, high-priced consulting firms, and IT professionals. These are the very people who have a vested interest in proffering big data solutions – even if they only have a shallow understanding of what the data represent. In those circles there is a tendency to think that if the data sets are large enough, sophisticated algorithms can somehow smooth out flaws in the data. Yet the old truism still holds: garbage in garbage out.
Huge numbers, per se, may awe the innumerate and methodologically challenged, but honest social scientists have long recognized that quality and critical understanding are what really counts. For example, a relatively small scientific survey, a tight, well-designed experimental design, or a rigorous, clearly defined accounting process provide validity that a conglomeration of user reviews and click data cannot deliver. Why so?
Let’s first look at what much of big data is based on. For sure, some data are obtained via valid techniques with clearly defined outcomes and caveats. Unfortunately, those methodologies can be expensive, so they are often supplanted by cheaper, less rigorous approaches. One such approach is what is known as a convenience or opt-in survey. Typically conducted online, these surveys appear in the form of a pop-up or as an embedding link.1
These unscientific approaches typically lack basic validity. Rather, the findings reflect an amorphous aggregation of people who happen to be visiting a given web page at a particular time. Google surveys, for one, are very quick, inexpensive and beloved by techno geeks, but, in the end, you get what you pay. A legitimate sample should represent a given population based on sampling frame and response rate, which opt-in surveys cannot provide.
The key here is to sample a representative audience, rather than people who happen to be on-line, or like to air their opinions or have an ax to grind. In addition, the response rates for pop-up surveys are absurdly low, so it’s hard to evaluate how representative their findings may be. As Butch said to Sundance, the client should ask their consultants, “Who ARE those guys?” Opt-in surveys, at best, may crudely identify major trends – providing that the client is willing to foot the bill for a tracking study – and also can also suggest there is a major issue worth exploring more rigorously.
How about user reviews? Note that they are usually based on customer ratings elicited right after purchase. The most relevant issues of usability and product reliability are not even factored into the equation. The purchaser also is typically comparing a brand new product with a much older and often poorly performing model. (My new 42” Sanyo TV may seem great compared to my old six year old 34” Toshiba.) That is why product user reviews tend to be so positively skewed. Finally, and this no small problem, many reviews are bogus, provided by outside firms for a fee. Vendors claim to scrutinize these reviews, but, like NSA’s protocols, one ultimately needs to take them at their word. User reviews sometimes provide the best data one can find (e.g., Trip Advisor for non-chain hotels or restaurant), but one may be safer viewing them for specific comments rather than for their summary ratings.
Other metrics involve click data on a website, which may be more indicative of placement on a web site than anything else. If a link is prominently and explicitly featured, it will get more page hits. If a page requires complex navigation to reach, it will garner fewer hits, especially if the search tool is flawed. Here’s a real life example from my experience at Consumer Reports. Many non-product ratings (supermarkets, airlines, insurance, etc.) attained exceptionally high readership scores. On the website, ConsumerReports.org, these stories are buried and invisible to many potential readers. Note that when those stories briefly appeared on the home page they were extremely popular. Rather than look at the pattern analytically, the big data decision was to focus on IT-based metrics such as click data, which were seen as both “objective” and “real time” despite their obvious flaws.
Another popular yet overrated methodology is the focus group, a moderated discussion among selected participants on a particular topic. Focus groups are usually comprised of people selected for some basic demographics and a roughly defined unifying theme such as in the market to buy a car or does online research on health care. Note that these are people have both the time and inclination to spend a couple of hours on a topic for a small fee and a meal. Second, unless the moderator is very adept, the prejudices of the moderator or highly opinionated participants often exercise undue influence. Clients often latch onto the opinions of participants with whom they agreed, thereby drawing suspect conclusions.2 One valid use of focus groups is to help clarify issues for later quantitative work. Another valid use of focus groups is when the participants possess true expertise or other qualifications. For example, I conducted a focus group with electric engineers on microchips and another among senior directors and VPs at commercial banks on issues involving online banking.
Number crunching – a la big data – without appropriate history or solid methodology has very limited utility. Human behavior is not akin to physics, and numerical positivism is often fatally flawed. Too much analysis is largely ahistorical. “Real time data” is another data cliché much lauded today as the holy grail of research. Yes, up-to-date data are invaluable, but good data analysis requires thought and perspective. What does one make of the number of tweets or Face Book posts for Justin Bieber in March 2014?
Even scientific survey research needs to acknowledge historical precedents. I recall an ongoing Gallup survey that asked were the biggest concerns Americans were facing. Most of the time various economic issues were volunteered; however, when issues like drugs, HIV, or crime dominated the news, those issues which seemed paramount at the time quickly faded in the public’s eye. Thus “real time data” without context can be both shallow and misleading.
Another key point: Watch for bias. One reason that people highly rate expensive new purchases is that they don’t want to admit that they may have made a mistake (cognitive dissonance in social science parlance). Sometimes ideology obscures opinion. Careful question creation will avoid many of the pitfalls. Questions posed in terms of “consumer protection” will tell a different story than questions framed as “government regulation”. It is often assumed that “anyone can write a survey”, but such naiveté will provide bad results.
Different data tell different stories. What are the strength and limitations of each dataset? Blind number crunching obscures reality, and no amount of sophisticated statistical techniques can produce valid conclusions unless the data collection methods are evaluated and found sound. If two different analyses tell different stories, the object is to see why. Are they measuring the same thing?
At Consumer Reports we found sometimes a car model’s rating from lab tests did not jibe with the survey results. Both methods are valid, but the former are predicated on measurement of performance based on lab test while the survey reliability is based on respondents reporting that product broke within a given time frame. Both measures had strength and weaknesses. A product can perform very well, yet have undistinguished reliability, and vice versa. Both sets of data are presented, but not aggregated. Doing so may delight the wonks by providing a “simple” measure, to do so will obscure reality and will do the client/audience a major disservice.
Another example: A number of years ago I was asked to represent Consumer Reports at a health care conference sponsored by Kaiser Family Foundation. Most of the major health care research firms as well as several major employers attended. One of the goals was to ascertain whether a basic metric evaluating health care would be possible. The general consensus was that goal was illusory because the data were far too complex and multivariate to do so. In the most simplistic terms, you can’t have red and blue and say the answer is purple. So while big data may contain reams of information, it cannot be boiled down to simplistic conclusions.
So here’s my advice. All datasets, large and small, have strengths and limitations. Bigger does not necessarily mean better. You will learn more from a well-constructed small set of data than from a less robust but large one. And while sloppy data analysis can obscure the value of even the best data, even the most sophisticated data analysis cannot rescue meaningless data. Statisticians and web wonks are not members of a priesthood. Don’t assume they have all the answers. Like the patient who is told that surgery is necessary, you may want to get a second opinion. After all, it’s your business and you should not hand over key decisions to number-crunchers who might have little understanding of your industry, its dynamics, or your customers.
Mark Kotkin, PhD, retired from Consumer Reports after 27 years. He worked in their survey division, most recently as Director. He was responsible for all published survey-based content and served as a methodologist on several organizational teams. He managed the Annual Questionnaire, the largest US survey outside the Census. Previously he had conducted market research on major corporations for a major research firm based in NYC. He currently consults for private clients.
Photo by Fernanda B. Viégas
1 Note that scientific surveys can be done online provided there is an appropriate methodology. Consumer Reports and GfK are two organizations who have done so.
2 A related approach—in-depth interviews—avoids some of those pitfalls, but not the issue of representativeness.
The author focuses on the
The author focuses on the risks of unscientific survey or 'voting ' data which is wise. But most real uses of big data are conducted by companies who rely on true consumer voting data. Specifically, what did consumers pay cold cash for and when and where did they do it? This is vastly better information than that gleaned from the best survey or even a political election because it reflects the real tradeoffs of real people making real choices. By contrast a vote for Obama or Romney does no such thing. It simply reflects the ease by which voting by mail can be done.